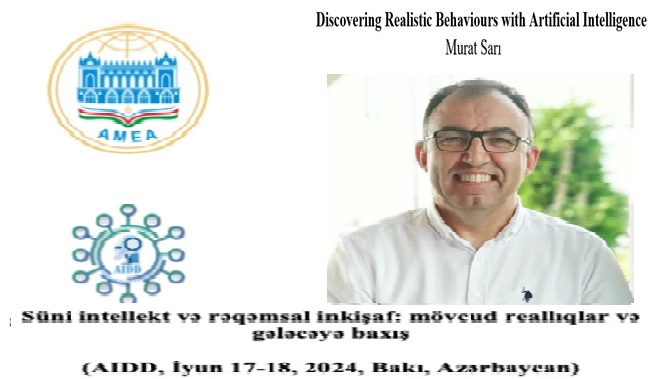
This presentation highlights the impact of artificial intelligence (AI) on modeling and predicting realistic behaviors in complex systems. Advanced techniques, including physics-informed neural networks and innovative approaches, are introduced to address challenges such as nonlinearity and uncertainty. The session concludes with a vision to explore AI’s potential in broader scientific and practical applications.
This presentation delves into exploring realistic behaviors using artificial intelligence (AI), focusing on advanced modeling approaches to analyze and predict complex systems. By examining the interplay of mathematical modeling and experimental studies, the talk aims to demonstrate how AI enhances understanding of real-world processes characterized by nonlinearity, uncertainty, and stochasticity.
Key topics include the distinction between forward and inverse problems, the role of continuous, discrete, simulation, and AI methods, and the challenges of modeling real-world systems. AI's flexibility and capability to capture intricate behaviors are emphasized, with examples highlighting nonlinear advection-diffusion equations and other complex phenomena. These examples showcase how empirical and theoretical models complement each other to provide numerical insights and actionable predictions.
The discussion also introduces cutting-edge AI applications, such as discretization-free approaches and physics-informed neural networks, emphasizing their role in solving high-dimensional and nonlinear problems. By comparing traditional computational methods with AI-driven techniques, the presentation underscores the transformative potential of AI in addressing the limitations of conventional models.
The session concludes with a vision for future developments, encouraging the refinement of AI methodologies to uncover deeper insights into realistic behaviors, thereby advancing scientific inquiry and practical applications across diverse domains.
.
https://aidd.anas.az/